A few housekeeping items before jumping into the article:
I will be at the ACP Conference early next month (you can message me on the ACO Conference app if you are there and want to meet up).
I was invited for an XPC Talk by Paulius Mui, MD, who runs a primary care innovation community with my favorite tagline, “a group of misfits in healthcare who like to learn together.” You can check out his work on his website X=Primary Care.
The YouTube video of my talk is embedded below.
With that out of the way, let's move on to today’s article.
An ounce of prevention is worth a pound of cure.
—Benjamin Franklin.
In my last article, “What is a disease?” we looked at the complexity and nuances involved in defining a disease.
In this article, take a deep dive into the complexity and nuances of screening for disease, explore how the benefits of screening are exaggerated, and its relationship to profits in healthcare.
An ounce of overdiagnosis is worth pounds of profits.
—Claude 3 Opus
This is a complicated topic, and I want to make 2 points before getting into the details:
I am not advocating against screening, including cancer screening. Cancer screening should be a choice, not a quality measure.
Please talk to your doctor first before deciding to forego any screening.
The video version of this article is embedded below and on my YouTube Channel.
Audio podcast and video versions are also available on the Podcasts Page.
Purpose of Screening
The central purpose of screening is to:
Identify disease early.
Start treatment early to reduce the probability of symptoms, morbidity, or mortality.
Principles of Screening
Wilson et al. published the basic principles of screening back in 1968.1 I have attempted to summarize and modernize the relevant parts of this handbook below. According to this handbook, the principles of screening are:
The condition sought should be an important health problem.
There should be an accepted treatment for patients with recognized disease.
Facilities for diagnosis and treatment should be available.
There should be a recognizable latent or early symptomatic stage.
There should be a suitable test or examination.
The test should be acceptable to the population.
The natural history of the condition, including development from latent to declared disease, should be adequately understood.
There should be an agreed policy on whom to treat as patients.
The cost of case-finding (including diagnosis and treatment of patients diagnosed) should be economically balanced in relation to possible expenditure on medical care as a whole.
Case-finding should be a continuing process and not a "once and for all" project.
These principles are meant to guide decisions about whether or not to screen, and they emphasize the need to balance the potential benefits and harms of screening. The three principles in bold above are especially fraught with nuances and bias that can tilt the balance from benefit to harm.
What Makes a Good Screening Test?
Before we examine the nuances and biases of screening, let’s examine what makes a good screening test. Several criteria are used to decide which medical test to use for screening.
Validity
Validity refers to the test’s ability to accurately distinguish between individuals who have a disease and those who do not. It is typically assessed using Sensitivity and Specificity.
If you are unfamiliar with Sensitivity/Specificity/PPV/NPV, I recommend the excellent overview at Sensible Medicine—Improving Your Critical Appraisal Skills #4.
Reliability
Reliability refers to a test’s ability to consistently produce the same results when repeated, generally under the same conditions. It is sometimes also called reproducibility or precision.
Different times: BP readings may be different in the morning and evening
Different observers: BP results may differ depending on the patient's expertise in taking their BP.
Different settings or conditions: BP may be different in a doctor’s office vs at home.2
Yield
The yield is the number of new people found to have the disease as a result of the screening program. Yield is related to disease prevalence in the population and access to medical care. The highest yields will be obtained from screening for a highly prevalent condition in a population that has minimal access to medical care.
On the other hand, when people have access to medical care, fewer new diseases are likely to be discovered through screening, as the disease will be found as part of routine medical care—this is called the yield paradox.
Furthermore, most screening programs cast a wide net, tolerating more false positives to avoid missing a diagnosis. As a result, when a screening program is implemented in a region with good access to medical care, a higher percentage of "new diseases" identified through screening are more likely to be false positives.
For example, the “Depression Screening and Follow-Up Plan” quality measure is more useful when people lack access to medical care. Mandating PCPs to conduct a mass screening campaign for all their patients with medical access is more likely to yield false positives. I discussed these issues in my prior article, “The Depressing Reality of Depression Screening.”
Cost Effective
The screening test should be affordable and provide good value when applied to large populations. This also raises the question of who benefits—the entire population, a select number of people, providers, or manufacturers of screening tests?
Furthermore, screening for a disease often fails to account for the additional time required, particularly in primary care, to conduct screening and to document and report it for quality measures.
Additional criteria for deciding if a test should be used as a screening test include acceptance by people, the simplicity of performing the test, and the availability of follow-up services.
The Disease Definition Dilemma
Before we can recognize a latent or early symptomatic stage, we need to define the disease.
I discussed the role of the “natural history of disease,” or my preferred term, “biologic pathway,” in defining disease in my last article, “What is a disease?”. The central questions for screening then are:
Where in the biologic pathway do we screen? In other words, how do we define the latent stage?
How long will it take for the disease to progress from the latent stage (when screening is performed) to clinical symptoms?
Do we have a treatment that works at the latent stage?
What are the side effects of treatment, and how will they affect the quality of life?
Defining Latent Stage
Physiologic variables, such as BP, blood sugar, and even cancer cells, are continuously distributed around the mean, conforming to a normal or skewed normal curve. This distribution is either bimodal or unimodal.
Bimodal Distribution
If the distribution of physiologic variables is bimodal, there will be an overlap between individuals considered normal and those with the disease.
Take cancer as an example. During normal cell turnover, many “abnormal cells” are produced in the human body, which could be classified as cancer cells under a microscope. In healthy individuals, the immune system eliminates these abnormal cells before they can cause disease. However, when these cells evade the immune system, they can lead to symptoms that we recognize as cancer.3
This creates a significant overlap between individuals who are normal (i.e., have cancer cells but no symptoms) and those who have symptomatic cancer (represented by the shaded area in the first graph below).
In this case, one could argue that the latent stage consists of individuals without symptoms who fall under the shaded area of the “normal” curve, excluding those with the disease. These individuals have cancer cells that may eventually cause symptoms. Current screening strategies aim to identify all such individuals, operating under the assumption that all cancer cells are harmful. This approach overlooks the fact that, for the majority of people, these cells will never progress to cause symptoms (see biases in cancer screening below).
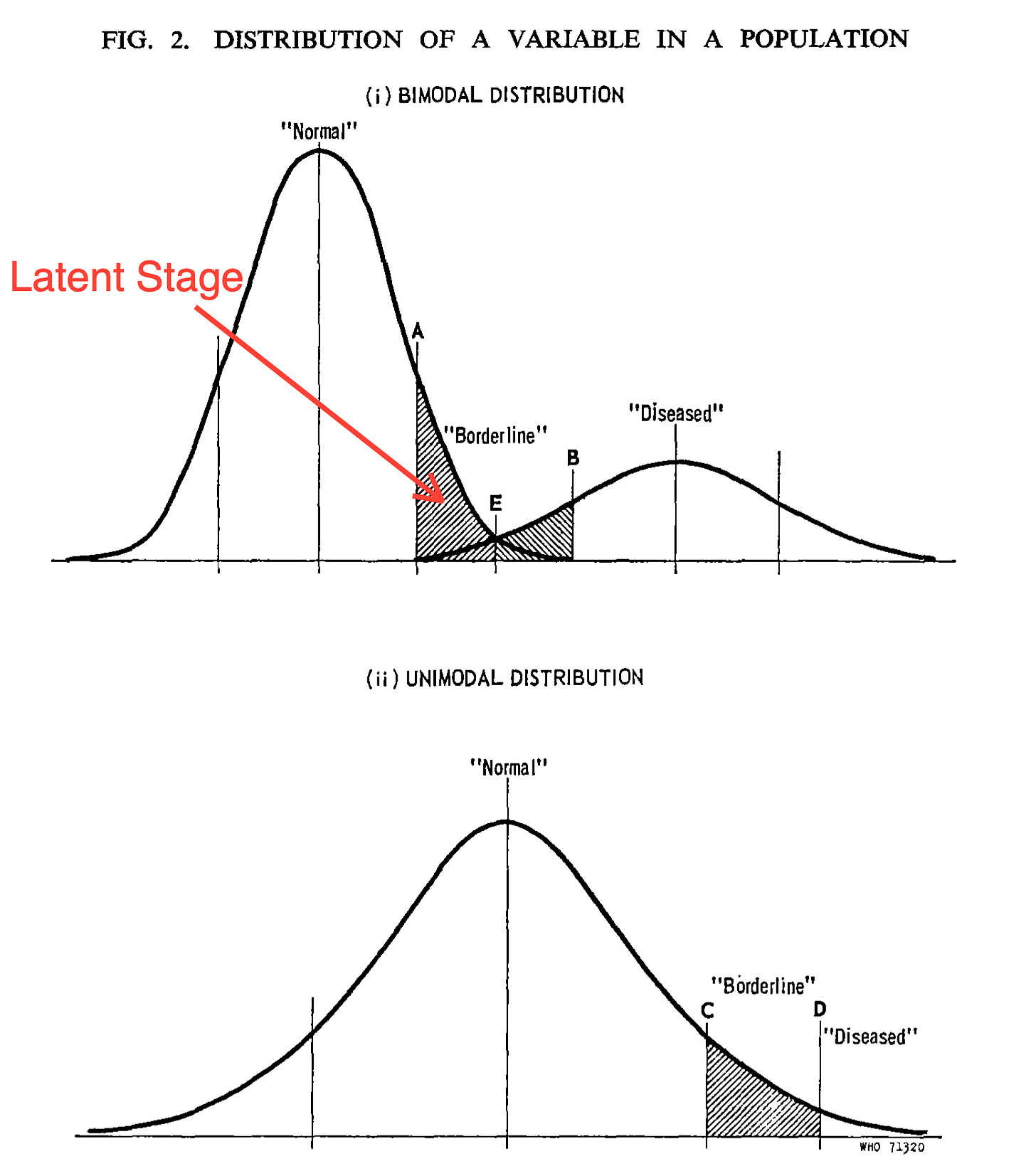
Unimodal Distribution
In an unimodal distribution, there is no clear distinction between “normal” and "diseased." Instead, clinical studies are used to establish an artificial cutoff point, which classifies everyone above the threshold as diseased. For example:
Fasting blood sugar > 126 mg/dL = Diabetes.
BP > 140/90 = Hypertension
Dysplastic cells = Normal cell repair produces dysplastic cells, which are always cleared by the body’s immune system. At what point during the unimodal distribution do we define these dysplastic cells as “cancer,” i.e., how many dysplastic cells do we need to classify “cancer.”
The challenge with determining a cut-off point in an unimodal curve is the law of diminishing returns if we keep lowering the cut-off value:
Lowering the cutoff value may classify more healthy individuals as diseased, leading to unnecessary treatment and lower quality of life.
Once these “normal” individuals are diagnosed with a disease, it may also increase their disability and life insurance premiums.4
Lies, Damned Lies and Statistics
Okay, that headline is a little over the top, but there is some truth to it. There are statistical techniques that can give the appearance of efficacy to a test when, in fact, the test is completely useless.5 Also, I will use cancer screening as an example to lay the groundwork for my next article.
Overdiagnosis Bias
In the case of a bimodal distribution of disease, we use sensitivity/specificity to decide on a screening test. Since there is no perfect test, we have to make tradeoffs. If we decide on a screening test that has higher sensitivity, we will find more false positives. For example, the original studies of breast cancer screening were based on “film-based” mammograms. The mammograms in use today are much more sensitive, with more false positives.
In the diagram below, you can see the 5-year survival doubles (increases from 40% to 80%) when you increase the screening of a test without any change in patient outcomes.
Lead Time Bias
Lead time bias occurs when earlier detection of a disease (e.g., through screening) appears to improve survival time, but in reality, it only increases the time the disease is known without altering the actual outcome or lifespan. In other words, it creates a false impression of effectiveness without true benefit.
The diagram below demonstrates an example of cancer screening done at age 60 that did not prolong the individual's life but statistically increased his/her 5-year survival rate.
Length Bias
Length time bias occurs when slower-progressing, less aggressive diseases are more likely to be detected during screening, giving the illusion of better survival rates, even though the screening doesn't change the natural course of the disease.
The Paradox of Treatment
As medical treatment for a disease improves, the benefits of screening diminish, and the risk of harm from treating false positives increases. We have much better treatments for breast and prostate cancer than 30-40 years ago when the original studies for screening were done. These modern treatments have dramatically improved survival rates, with the unintended side effect of possibly reducing the benefit of screening.
The Paradox of Screening
When healthcare systems implement screening based on limited or preliminary data that early detection improves outcomes, it can become culturally ingrained, almost like folklore. This cultural acceptance can make it ethically challenging to conduct studies comparing treatment with placebo once an early diagnosis is made through screening.
Imagine attempting to conduct a study today comparing mammograms to a placebo.6 Digital mammograms used today are more sensitive than older film-based mammograms. The higher sensitivity will detect more abnormalities, with a higher likelihood of false positives leading to net harm in the screened population.
However, because mammography is already widely accepted as a standard screening tool, conducting such a study to evaluate its true benefit versus harm would likely be considered unethical. This is due to the cultural and medical assumption that mammograms save lives, even though newer technologies may shift the balance between harm and benefit.7
E.g., In 2012, when USPSTF issued a Grade D recommendation against PSA-based screening for prostate cancer, many patients and advocacy groups were concerned that the recommendation would lead to underdiagnosis and potentially worse outcomes for men who might benefit from early detection.8
The Pound of Profits
Screening programs, while intended to improve public health, are often influenced by financial incentives. For example, more sensitive mammograms lead to more false positives and unnecessary treatments—generating higher revenues. Or, using a sensitive screening questionnaire like PHQ-9 will ensure that more people will be prescribed antidepressants.
All healthcare entities, including doctors, profit from widespread screening, even when the evidence supporting its benefits is limited. However, the role of individual doctors is much more complicated:
There is growing evidence of financial conflicts of interest among physicians who write guidelines.9
Most doctors rely on guidelines and don’t look at the underlying evidence themselves. Therefore, guidelines have a significant impact on clinical decisions made by doctors.
Doctors are humans too, and have the same fear of dying. And we have seen loved ones succumb to diseases, including cancer. This means we fall prey to the same beliefs that guideline writers publish—and undergo the same screening tests with limited benefits.
Most doctors are employed and do what they are told by the large consolidated healthcare institutions they work for, i.e., generate profits.
And by the way, most guideline authors work for these large consolidated healthcare systems!10
Once a medical test is culturally ingrained and recommended by guidelines, it is generally considered medical malpractice if doctors don’t perform the screening test.
I discuss this phenomenon in my prior article, “The Quality of Quality Measurement.”
Many screening tests are also quality measures (e.g., breast and colon cancer screening), which are directly linked to penalties for individual doctors—financial, reputational, and medico-legal.
Most people equate cancer with a death sentence, even though treatment has improved dramatically. Aggressive advertising by health organizations and medical test manufacturers fuels this anxiety further, creating demand for their products—A person’s peace of mind is healthcare’s profit center.
Conclusion
While screening has repeatedly been touted as saving lives by detecting diseases early, it also carries significant risks, including overdiagnosis and false positives, which harms patients. A consolidated medical-industrial complex exploits these screening tests by advertising, influencing medical guidelines, and recommending more sensitive tests with higher false positives, leading to higher profits.
Remember the old adage:
A stitch in time saves nine.
In medicine, sometimes early stitches may tear the fabric of health while filling the coffers of the medical-industrial complex.
Up Next
This article laid the groundwork for understanding the pros and cons of screening for disease. The next article will examine the Colorectal Cancer Screening Quality Measure.
If you liked this article, please consider sharing it.
Wilson, J. M. G., Jungner, G., & Organization, W. H. (1968). Principles and practice of screening for disease. https://iris.who.int/handle/10665/37650
This last point is generally not a standard definition of reliability but is very important in clinical medicine, as the BP example demonstrates.
Needless to say, this is an oversimplification of cancer biology.
Interestingly, BP cutoffs were initially made by insurance firms conducting actuarial analyses to price life insurance.
Whelton, P. K. (2019). Evolution of blood pressure clinical practice guidelines: A personal perspective. The Canadian Journal of Cardiology, 35(5), 570–581. https://doi.org/10.1016/j.cjca.2019.02.019
Wegwarth, O., Schwartz, L. M., Woloshin, S., Gaissmaier, W., & Gigerenzer, G. (2012). Do physicians understand cancer screening statistics? A national survey of primary care physicians in the United States. Annals of Internal Medicine, 156(5), 340–349. https://doi.org/10.7326/0003-4819-156-5-201203060-00005
The WISDOM trial is currently testing the efficacy of mammograms with the control arm being a “risk-based approach” instead of “no-screening.
Scherer, L. D., Valentine, K. D., Patel, N., Baker, S. G., & Fagerlin, A. (2019). A bias for action in cancer screening? Journal of Experimental Psychology. Applied, 25(2), 149–161. https://doi.org/10.1037/xap0000177
Schwartz, L. M., Woloshin, S., Fowler, F. J., & Welch, H. G. (2004). Enthusiasm for cancer screening in the United States. JAMA, 291(1), 71–78. https://doi.org/10.1001/jama.291.1.71
Harris, G. (2011, October 6). U.S. Panel Says No to Prostate Screening for Healthy Men. The New York Times. https://www.nytimes.com/2011/10/07/health/07prostate.html
Mooghali, M., Glick, L., Ramachandran, R., & Ross, J. S. (2023). Financial conflicts of interest among US physician authors of 2020 clinical practice guidelines: A cross-sectional study. BMJ Open, 13(1), e069115. https://doi.org/10.1136/bmjopen-2022-069115
It may appear from my remarks that I am throwing the guideline authors under the bus. I am sure that they are trying their best to do the right thing for the population. But there is always the ever-present unconscious bias!
This was interesting and informative. Thank you for putting this together.